People
Dr Tim Lucas
Lecturer
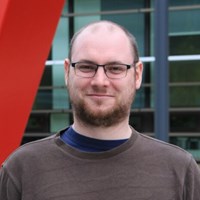
School/Department: Population Health Sciences, Department of
Email: tim.lucas@leicester.ac.uk
Profile
- Geostatistics
- Machine Learning
- Epidemiology
I am a lecturer in biostatistics. My interests lie in problems involving observational data and often with a focus on prediction. This has involved work on geospatial models, machine learning models and disaggregation regression models as well as how to combine models from different modelling paradigms such as combining Bayesian statistics and machine learning models.
I studied an MBioSci in Zoology at the University of Sheffield followed by a PhD in disease ecology (bats and zoonotic diseases) at University College London. I then joined the Malaria Atlas Project for a postdoc developing disaggregation regression models for predicting malaria incidence. After a short post-doc studying neglected tropical diseases and one year as a research fellow at Imperial College studying air pollution I joined the Department of Population Health Sciences as a lecturer.
To say hello on twitter see either of my twitter handles.
My github webpage has more content as I have more control over it.
I host my code on github.
My publications are on google scholar.
Research
In many cases we have low resolution disease data (daily symptom reports weekly hospital episodes etc.) but high resolution covariate data (minute-resolution air pollution data for example). I am continuing to develop disaggregation regression models to appropriately handle these data. These new methods might account for aggregation of risk over space, time or human activity. I am working on applications in zoonotic diseases, air pollution, asthma and malaria.
During my time at the Malaria Atlas Project I developed methods for estimating high-resolution (5x5 km pixel) malaria incidence from low-resolution (county level for example) routine surveillance data from hospitals. We created the first global spatio-temporal maps of Plasmodium falciparum and P. vivax. We also developed methods for combining low-resolution routine surveillance data with prevalence survey data using joint-likelihood models and machine learning models. We also performed simulation studies to evaluate when these methods fail and made software available for other researchers to use and applied these methods to SARS-CoV-2 data.
During the COVID epidemic I was involved in modelling the effectiveness of contact tracing and evaluating how important adherence and delays are.