Professor Ivan Tyukin
Professor of Applied Mathematics
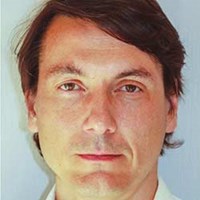
Profile
Professor Ivan Tyukin is an expert in the mathematical foundations of Artificial Intelligence (AI) and learning systems, Machine Learning, mathematical modelling, adaptive systems, inverse problems with nonconvex and nonlinear parameterization, data analytics, and computer vision.
Professor Tyukin has been awarded a prestigious Turing AI Acceleration Fellowship to lead innovative and creative AI research with transformative impact. He is a member of the IFAC Technical Committee on Adaptive and Learning Systems, an Editor of Communications in Nonlinear Science and Numerical Simulations, and is a member of the All Party Parliamentary Group on AI's data governance task force which examines the economic, social, and ethical implications of AI. He has a broad network of academic and industrial collaborators includes strategic UK sectors such as public safety and security, health technologies, space and Earth Observation, and manufacturing.
Professor Tyukin’s recent work focuses on creating a theory and practice for developing AI systems that are provably robust, resilient, certifiable, trustworthy, human-centric and data-driven. The theory will enable the creation of new-generation AI systems which are certifiably stable, secure, adaptive and maintainable. These systems will be prepared to handle the challenges of adversarial attacks and data inconsistences, uncertainties, and bias inherent within any empirical data. This will enable new gold standard methods and tools in tasks that are currently heavily reliant upon non-deterministic human input to have long-term transformation.
Research
My long-term research interests revolve around challenges of creating theories, methods, and algorithms underpinning creation and understanding of machine intelligence, adaptation, and learning as well as helping to reveal their fundamental limitations. Development of these theoretical tools requires combination of techniques from different areas spanning concentration of measure theory, statistical learning theory, analysis, modelling and synthesis of fragile, nonlinear, chaotic, meta-stable dynamics, adaptation and adaptive control theory, synchronization (stable and critical), biologically-inspired systems for processing of the visual information, computer vision, networks of interconnected dynamical systems, analysis of dynamics of the spiking neuron models, their properties and possible functions, algorithms for machine learning and data analysis in high dimensions. For the sake of simplicity, I organized these different lines of inquiry into the following specific challenges I am addressing at the moment:
- Provably Resilient, Trustworthy, and Robust Artificial Intelligence. Discovering general principles and fundamental understanding of how to build certifiably safe, quantifiable, and trustworthy AI systems for the benefit of people.
- Machine Learning and Data Analysis in High dimensions. Exploiting measure concentration effects for development and analysis of efficient algorithms for machine learning and data analytics in high dimensions.
- Processes and Mechanisms of Adaptation in Complex Nonlinear Systems. Systems with nonlinear parametrization, unstable target dynamics, non-dominating (non-majorating, gentle, non-dominating) adaptation
- Synchronization in Nonlinear Dynamical Systems. Global, partial, intermittent synchronization in the ensembles of linearly and nonlinearly coupled nonlinear oscillators. Study of connectivity-dependent synchronization. Adaptive and unstable, multi-stable, alternating synchrony.
- Optimization algorithms for nonconvex and nonlinear problems. Parameter estimation and inverse problems involving systems of ordinary differential equations with nonlinear in parameter right-hand side.
- Analysis of dynamical systems with unstable and semi-stable attractors. Extension of the method of Lyapunov functions for systems with weakly attracting (in Milnor sense) invariant sets, first method of Lyapunov for non-hyperbolic equilibria, non-uniform small-gain theorems.
- Neuroscience and physics of neuronal cells. Principles of neuronal processing of information. Study of properties of the biological cells, analysis of their functions. Structural organization of the visual system, models system for robust and adaptive processing (w.r.t modelled uncertainties) of visual information.
Publications
S. Wang, M.E. Celebi, Y.D. Zhang, X. Yu, S. Lu, X. Yao, Q. Zhou, M.G. Miguel, Y. Tian, J.M. Gorriz, I. Tyukin. Advances in data preprocessing for biomedical data fusion: an overview of the methods, challenges, and prospects. Information Fusion, 76, 376-421, 2021.
S.J. Núñez Jareño, D.P. van Helden, E.M. Mirkes, I.Y. Tyukin, P.M. Allison. Learning from scarce information: using synthetic data to classify Roman fine ware pottery. Entropy, 23(9), p.1140, 2021.
A.N. Gorban, B. Grechuk, E.M. Mirkes, S.V. Stasenko, I.Y. Tyukin. High-dimensional separability for one-and few-shot learning. Entropy, 23(8), p.1090, 2021.
I.Y. Tyukin, A.N. Gorban, A.A. McEwan, S. Meshkinfamfard, S. and L. Tang. Blessing of dimensionality at the edge and geometry of few-shot learning. Information Sciences, 564, 124-143, 2021.
B. Grechuk, A.N. Gorban, I.Y. Tyukin. General stochastic separation theorems with optimal bounds. Neural Networks, 138, 33-56, 2021.
N.V. Brilliantov, H. Abutuqayqah, I.Y. Tyukin, S.A. Matveev. Swirlonic state of active matter. Scientific Reports, 10, 16783, 2020.
C. Calvo, I.Y. Tyukin, V.A. Makarov. Universal Principles Justify the Existence of Concept Cells. Scientific Reports, 10, 7889, 2020.
A.N. Gorban, V.A. Makarov, I.Y. Tyukin. High-Dimensional Brain in a High-Dimensional World: Blessing of Dimensionality. Entropy, 22(1), 82, 2020.
A.N. Gorban, V.A. Makarov, I.Y. Tyukin. Symphony of high-dimensional brain. Reply to comments on "the unreasonable effectiveness of small neural ensembles in high-dimensional brain". Physics of Life Reviews, 29, 115-119, 2019.
A.N. Gorban, E. Mirkes, I. Tyukin. How deep should be the depth of convolutional neural networks: a backyard dog case study. Cognitive Computation, 2019. https://doi.org/10.1007/s12559-019-09667-7.
Teaching
- MA2021/MA2022 - Differential equations and dynamics
- MA3077/MA7077 - Operations Research